Why AI wont steal our broadcast graphics jobs – but it might change them…
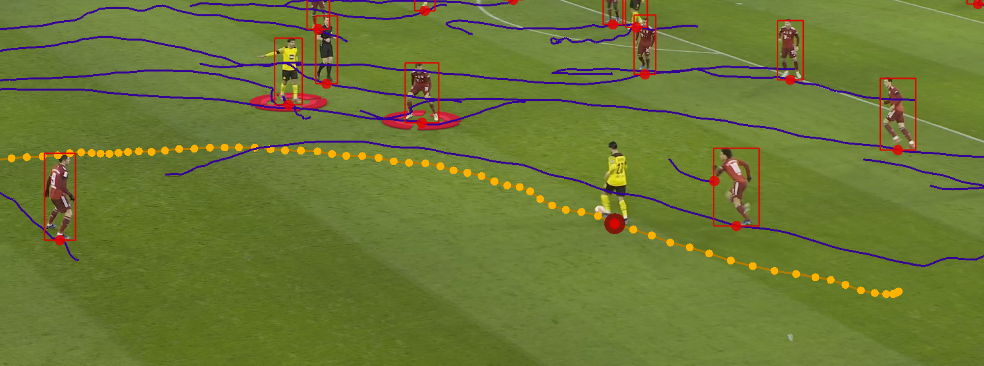
We have all seen the consternation in the media about the rising challenge of AI in a wide range of industries and the potential for mass job losses as a result. Should we be concerned that the same could happen to workers in the broadcast graphics sector? The trouble with these kinds of sweeping statements is that they cover such a broad set of roles that it becomes meaningless. To make informed comments we really need to address each niche within ‘broadcast graphics’ separately and look at what AI could do, or is already doing, to see how it affects the users involved. What is true for some areas may be very different for others.
Let’s start with telestration, aka sports analysis. Telestration is the process of adding lines, arrows and highlights to sports action. Although it is done for many sports, we most often see this on high value sports rights such as football. Its aim is to share insights about team tactics and to more deeply engage the viewers at home. By drawing the viewer’s attention to some critical action, or mistake, through the addition of pitch graphics, the broadcaster is attempting to impart inside knowledge about winning strategies.
Historically, the process for adding these lines onto the pitch, shafts of light above players, or arrows that illustrate the ball’s flightpath, was done manually by a human operator. But the task is often complicated because these elements need to be added over moving video. For example, the camera pans and the players run, but the graphics need to appear ‘stuck’ to the players or fixed to a position on the ground while all this is happening.
This requires a fair amount of technical skill for the operator to make a convincing analysis sequence. Although product developers aim to make it as easy as possible, it still requires time, effort and focus from the user. It remains very easy for an unskilled operator to do it badly!
This type of job role is prime territory for AI to move into. It’s normally a manual, laborious process that is prone to error, whereas AI can, for example, automatically identify players, and automatically attach graphics to them much more quickly and accurately than a human operator. AI can also be used to automatically calibrate a pitch (so graphics have the correct perspective), or track camera movements (where slight errors in how virtual graphics ‘stick’ to the pitch are very obvious to the human eye). Whilst it is possible to do manually, these are fiddly, tedious tasks and doing them well requires technical skill and concentration. In contrast, AI performs these tasks perfectly every time and considerably quicker. Data from one leading manufacturer found that their AI was between 60 and 150 times faster than the equivalent manual processes.
Some might argue reading this that AI is therefore de-skilling the role of the user, and potentially even eliminating them completely. What are they for if not to carry out these essential, but ultimately slightly fiddly jobs? Well I’d argue that the AI does not eliminate the operator or reduce the skill level at all. What it does do, is change the type of skills that the operator has to have, and I’d argue it changes it for the better.
Let’s back up for a moment and consider what skills we’d really like the operator to have. What are we asking them to do, really? It boils down to informing the viewers at home about the player’s skills, team tactics, management strategies and so on. It’s really about communicating an insider’s perspective to the layperson.
To achieve this, pre-AI, the operator needs to be adept at defining motion paths and configuring the software to achieve their aims. Post AI, the operator no longer needs those technical skills. Instead the job role can be defined around professional football experience. Someone who has been a football trainer, or player is now within scope of the role of telestrator, because handling the idiosyncrasies of controlling a technical software product is less important than understanding the game from the inside. What’s really important is the professional insight they bring to the role. Surely this is a great step forward for broadcasters and viewers alike?
There is evidence that this change is happening. Broadcasters might have a reasonable expectation regarding the technical ability of their staff. However, the same software packages are also used in professional teams to aid the coaching process rather than for TV broadcasting. The professional trainers need a system that supports their need to explain tactical successes and failures to their players in the training room. The available skills are different, and AI based telestrators let coaches focus on communicating their thoughts and insights to players more effectively because all the heavy lifting is taken care of.
We now see evidence that this process is going full circle. What may have started with the broadcasters, was enthusiastically grabbed with both hands by the teams themselves. The broadcasters now realise they could be using different types of operators with greater professional insights. Adopting AI based systems helps them in the battle for viewing figures.
Where is all this leading you may wonder? What’s clear is that we are only at the start of the AI journey. The next stage of AI telestration development is already underway in leading products and will move beyond the current ‘simple’ level of telestration. The next level will see more advanced computation deployed to instantly identify the different teams and be able to understand their shapes and formations to be able to impart ever greater insights to viewers at home.
I hope this has made the case that just as previous technology changes have led to evolution rather than disaster, and so it will be for AI. No doubt each segment is different and the changes will be unique to each type of work, but there will be plenty of opportunities as familiar systems continue to evolve and embrace AI.